2. Distributed Computing¶
This code has been tested on NimbleBox.ai instance with two Nvidia-T4 cards.
In this section we will see how you can train 1Bn+ parameter models directly from gperc
using GPipe
that allows model parallelism while avoiding the bottleneck that comes with using large batches by spliting
the compute for each batch in mini-batches so devices have higher utilisation.
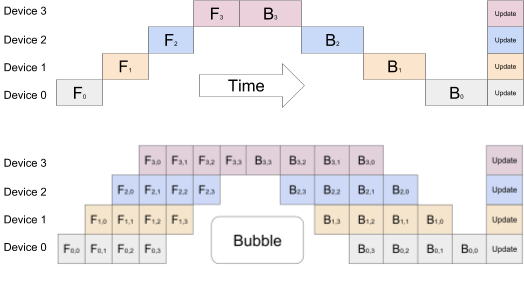
Some reference papers:
1. torch GPipe: This paper extends the original Gpipe for torch.
2. The code from above paper was added into the pytorch
and
this blog has tutorial for it.
Using GPipe
¶
I won’t go into details of why GPipe
was built and how pytorch
handles it internally but rather go
over the coding decisions.
All the functions take in dedicated keywords in the
forward()
method because sending ina tuple that can then be split requires serious modification to thepytorch
source code that can be a tiresome and tedious process. Example:
# forward method for Embeddings Module
def forward(self, input_array, attention_mask=None, output_array=None)
Now the
attention_mask
andoutput_array
can beNone
but when usingPipe
pytorch consumes only tensors and so I have added this weird quirk where you can send in tensors with same first dimension with values-69
for ignoringattention_mask
and-420
for ignoringoutput_array
. Yes very childish, I know. So in the script you will see code like this:
# output_array needs to be set None so be pass tensor with values -420
model_input = (
inputs.cuda(0),
attn_mask.cuda(0),
torch.tensor([-420. for _ in range(inputs.shape[0])]).cuda(0)
)
You will need to experiment with values
chunks
(total number of chunks to break the input into) andpartition_len
(number of modules on each chip). Returned attentions list also is chunked ie.
chunks = 16; batch_size = 32
output, attentions = model(*model_input)
# number of attentions == number of chunks
len(attentions) # 16
# batch size of attention layer is batch_size / chunks
attentions[0][0].shape[0] == 2